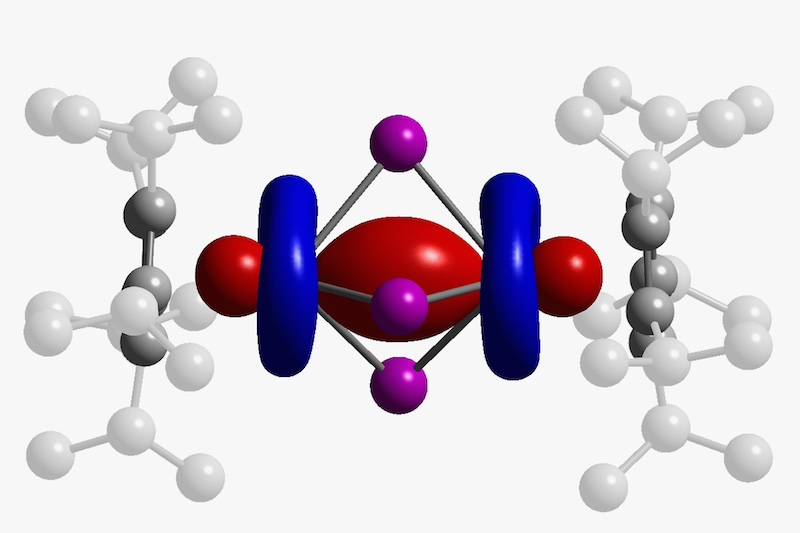
Tokyo university finds way to use deep learning to predict ‘exciting materials’
The method identifies magnetic materials solely based on their crystal structure, eliminating the need for time-consuming experiments and simulations
Single-molecule magnets (SMMs) are exciting materials. In a recent breakthrough, researchers from Tokyo University of Science have used deep learning to predict SMMs from 20,000 metal complexes.
The predictions were made solely based on the crystal structures of these metal complexes, thus eliminating the need for time-consuming experiments and complex simulations.
As a result, this method is expected to accelerate the development of functional materials, especially for high-density memory and quantum computing devices.
Synthesizing or studying certain materials in a laboratory setting often poses challenges due to safety concerns, impractical experimental conditions, or cost constraints.
In response, scientists are increasingly turning to deep learning methods which involve developing and training machine learning models to recognize patterns and relationships in data that include information about material properties, compositions, and behaviors.
Using deep learning, scientists can quickly make predictions about material properties based on the material’s composition, structure, and other relevant features, identify potential candidates for further investigation, and optimize synthesis conditions.
Now, in a study published on 1 February 2024 in the International Union of Crystallography Journal (IUCrJ), Professor Takashiro Akitsu, Assistant Professor Daisuke Nakane, and Mr Yuji Takiguchi from Tokyo University of Science (TUS) have used deep learning to predict single-molecule magnets (SMMs) from a pool of 20,000 metal complexes.
This innovative strategy streamlines the material discovery process by minimizing the need for lengthy experiments.
Single-molecule magnets (SMMs) are metal complexes that demonstrate magnetic relaxation behavior at the individual molecule level, where magnetic moments undergo changes or relaxation over time.
These materials have potential applications in the development of high-density memory, quantum molecular spintronic devices, and quantum computing devices. SMMs are characterized by having a high effective energy barrier (Ueff) for the magnetic moment to flip.
However, these values are typically in the range of tens to hundreds of Kelvins, making SMMs challenging to synthesize.
The researchers used deep-learning to identify the relationship between molecular structures and SMM behavior in metal complexes with salen-type ligands. These metal complexes were chosen as they can be easily synthesized by complexing aldehydes and amines with various 3d and 4f metals.
For the dataset, the researchers worked extensively to screen 800 papers from 2011 to 2021, collecting information on the crystal structure and determining if these complexes exhibited SMM behavior.
Additionally, they obtained 3D structural details of the molecules from the Cambridge Structural Database.
The molecular structure of the complexes was represented using voxels or 3D pixels, where each element was assigned a unique RGB value.
Subsequently, these voxel representations served as input to a 3D Convolutional Neural Network model based on the ResNet architecture. This model was specifically designed to classify molecules as either SMMs or non-SMMs by analyzing their 3D molecular images.
When the model was trained on a dataset of crystal structures of metal complexes containing salen type complexes, it achieved a 70% accuracy rate in distinguishing between the two categories.
When the model was tested on 20,000 crystal structures of metal complexes containing Schiff bases, it successfully discovered the metal complexes reported as single-molecule magnets.
“This is the first report of deep learning on the molecular structures of SMMs,” says Prof Akitsu.
Many of the predicted SMM structures involved multinuclear dysprosium complexes, known for their high Ueff values.
While this method simplifies the SMM discovery process, it is important to note that the model’s predictions are solely based on training data and do not explicitly link chemical structures with their quantum chemical calculations, a preferred method in AI-assisted molecular design.
Further experimental research is required to obtain the data of SMM behavior under uniform conditions.
However, this simplified approach has its advantages. It reduces the need for complex computational calculations and avoids the challenging task of simulating magnetism.
Prof Akitsu says: “Adopting such an approach can guide the design of innovative molecules, bringing about significant savings in time, resources, and costs in the development of functional materials.”
Main image courtesy of Science / AAAS