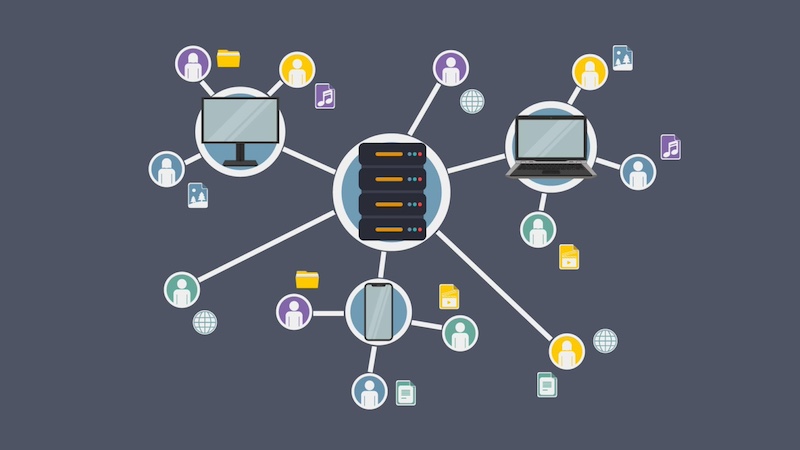
What Sets Data Quality and Data Integrity Apart?
At this point in the game, everyone knows just how important business data is to the overall success of an organization.
Not only does it feed analytical tools, but it acts as a pillar that businesses can leverage to make future predictions about risk, trends, and potential opportunities.
Yet, not all data is equal, with some forms being much more useful than others.
Bad data, data that is lacking in overall quality and integrity, costs businesses as much as $3 trillion each year, causing a huge expenditure of resources for very little in return.
To minimize the presence of bad data in an organization, most companies will employ a series of data initiates that dictate what data they capture, how it is structured, and the extent to which it is cleansed before moving into the data pipeline.
Despite the importance of these processes, many businesses still commonly confuse data quality and data integrity, assuming that they offer the same thing.
In this article, we’ll dive into the differences between data quality and data integrity, demonstrating how companies can get more from their data by understanding the terms and the processes behind them.
Let’s dive right in.
What is Data Quality?
Data quality is the overall measure that defines how useful data is to your business. High-quality data is any information that you can put to use for a positive business function, such as carrying out analytics or helping to guide you toward decisions.
The higher the quality of data, the more applicable it is to these functions, making it more valuable to your business.
Several core characteristics all feed into data quality, helping to improve its overall utility to businesses:
- Complete – Data that has major sectors missing or values that are not all in the right place would count as incomplete data. The more incomplete a data set is, the more holes there are, which then reduces its efficiency for data-driven processes. High-quality data is fully complete or almost 100% complete.
- Consistent – Data will often exist in two places at once across different subsets of that data. High-quality data ensures that the same data points are equal in all areas where they appear, demonstrating the consistency of that data set.
- Timely – Data is constantly shifting, self-actualizing, and morphing based on current findings. With that in mind, a business should check whether the data they’re using is representative of present information. Timely data refers to this characteristic, with data that is from the past failing on this point of data quality.
- Valid – Another core factor that contributes to data quality is the overall structure that it uses. Unstructured data or data in formats that are not compatible with a company’s analytical tools is much more costly and time-consuming to prepare and then use.
Overall, data quality represents the baseline characteristics of data that could or could not make data useful to a business.
However, a business that has high-quality data must also have the processes in place to use that data, which is where its integrity comes in.
What is Data Integrity?
Data integrity takes the use and interaction with data one step beyond its initial quality. Instead of its baseline characteristics, data integrity references all of the other aspects of a business that allow it to draw meaning from that data.
For example, if a business has the right data warehousing solutions that facilitate the storage and visibility of that data, then it will have a high degree of integrity.
Being able to trace data across its entire lifecycle with your business, and making sure to monitor any changes, ensures that has the characteristics needed to have high integrity.
Data integrity also monitors data across its deployment in every area of your business. If that business is altered, duplicated, or destroyed in the transfer, retrieval, and storage process, you should know about it.
Typically, data integrity will use methods like access controls, data validation techniques, and encryption to ensure it boasts high integrity.
What Sets Data Quality and Data Integrity Apart?
While both of these qualities describe certain aspects of data and a company’s interaction with data, they do not cover exactly the same thing.
You can differentiate data quality and data integrity by focusing on how they serve in three different arenas:
- Purpose – The purpose of data quality is to make data as easy to use as possible, boosting its utility in a business and helping them to feed it into analytical tools. On the other hand, data integrity refers to how trustworthy data is based on your control over it throughout your data pipeline, allowing you to prove its reliability.
- Application – High-quality data is vital in any data-related operation, being a priority in scenarios where you deal with analytics, visualization, reporting, and decision-making. Data with a high degree of integrity is important in business sectors where the reliability of data is key. For example, data integrity is essential in the healthcare system and financial records.
- Results – By using data with a high degree of integrity, businesses can demonstrate that no one has edited it during any other business process, allowing them to reuse data and store it for the long run. Alternatively, data quality ensures that you can utilize data in analytical processes, helping to align it structurally and formally with whatever tools your business uses.
Data quality and integrity are two crucial elements of data that allow businesses to get more from every data point they collect.
By focusing on running validation checks, limiting access to data, cleansing it before use, and creating a rigorous data quality framework, organizations can begin to improve their baseline levels of data management and reach new heights of utility.
Final Thoughts
Data quality and data integrity are two vital measurements that allow businesses to get more from their digital resources. By understanding the differences between these two aspects of data, businesses are more prepared to identify weaknesses in their data-cleansing process.
Over time, by focusing on improving quality and integrity separately, you can create highly efficient data processes.
Data is, above all else, the resource that allows businesses to thrive. When you begin to take additional care to make sure that your data is useable, effective, and well formatted, you’ll be able to draw more from this highly important resource.
While introducing changes that improve these characteristics of data won’t happen overnight, this process will have a dramatic impact on data-driven business operations when done successfully.
Investing in your own data is the best business decision you can make.