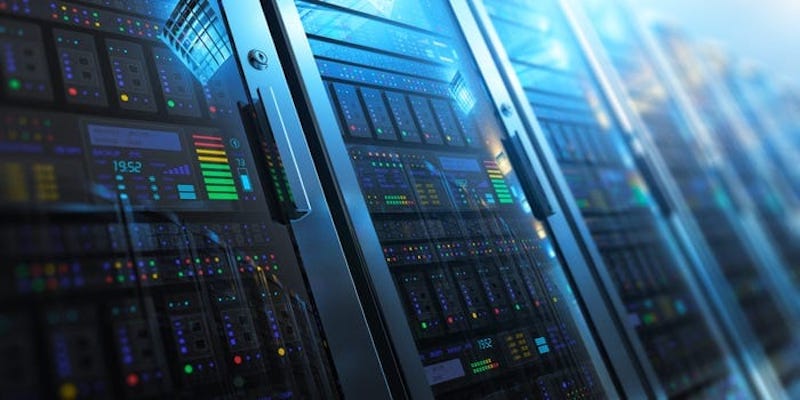
A Comprehensive Guide to Data Masking: Types and Techniques
In the present-day scenario, data breaches happen often. Companies must consider it with the utmost importance to protect their data and implement cutting-edge technologies for data protection.
Data masking is a technique that ensures security as it hides sensitive information in databases and apps to prevent theft. The original data’s format and usefulness are maintained.
This guide covers all you need to know about advanced masking techniques. We’ll discuss the types of available, essential methods like tokenization, best practices, examples, and future trends.
Whether you want to boost compliance or cyber resilience, this guide will show why robust data masking is crucial for modern businesses.
The Rising Importance of Data Masking
Data breaches and cyberattacks have been growing exponentially, making data security non-negotiable. According to Statista, the US alone faced 1,802 publicly reported data compromises in 2022, exposing the personal information of over 422 million individuals.
Clearly, as threat actors get more sophisticated, the stakes for data protection have never been higher. Multimillion-dollar fines for non-compliance pale in comparison to the reputational damage and loss of customer trust from a single breach. This propels data masking from a regulatory checkbox to an operational necessity.
In the financial sector, data compromises doubled between 2020 and 2022. With such alarming trends across industries, it’s evident that organizations can no longer rely on basic practices like siloed encryption. They require robust, advanced data masking integrated across the entire data infrastructure.
The Evolution of Data Masking
In the past, data masking mainly involved the retrospective application of basic encryption or substitution methods. These static, siloed techniques are no longer adequate given today’s threat landscape.
Modern data masking solutions must offer real-time protection and seamlessly integrate across on-premise, cloud, and hybrid environments. Leading techniques now include dynamic masking for on-demand access, format-preserving encryption to maintain data utility, and advanced tokenization.
As data volumes explode and cybercriminals leverage increasingly sophisticated attack vectors, data masking techniques must continue to evolve as well. Some innovations on the horizon include intelligent auto-masking using machine learning and quantum-resistant encryption.
However, while data masking technology has come a long way, the imperative remains unchanged – to enable controlled data use for operations and analytics without compromising security or compliance.
With the right data masking techniques in place, organizations can build cyber resilience while harnessing data’s full potential. However, to stay ahead of threats and regulations, businesses must take a proactive, layered approach to data security.
Advanced data masking techniques should form the core, complemented by strong access controls, network security, endpoint protection, and more. With a defense-in-depth strategy anchored by robust data masking, companies can confidently embark on digital transformation and safely thrive in the digital future.
Types of Data Masking
- Static Data Masking: This irreversible technique replaces sensitive data with aliases or anonymized data. It provides robust protection for large historical databases.
- Dynamic Data Masking: This masks data on the fly to enable access to real-time information without exposing actual sensitive data.
- Format-Preserving Encryption: This encrypts data while retaining its original format to prevent disruptions to dependent systems.
Understanding the use cases and trade-offs of each type is essential to building a tailored data masking strategy catered to your specific data landscape.
Key Data Masking Techniques
While the types of masking provide a high-level categorization, various techniques and algorithms make up their underlying implementation. Let’s delve into some of the most important data masking techniques:
- Tokenization: Tokenization replaces sensitive data with unique identification symbols or tokens. The token mapping is securely stored in a centralized vault. This prevents exposure of the original data.
- Data Anonymization: This technique randomizes personally identifiable information to make re-identification impossible. The anonymized data can safely be used for testing and analytics.
- Data Obfuscation: Obfuscation masks data by adding noise, shuffling, masking, etc., to preserve privacy without losing analytical value. It provides utility similar to anonymization but with greater data integrity.
Choosing techniques relies on data types, infrastructure, use cases, and regulatory needs. A blend of methods is often required for comprehensive data masking.
Implementing a Data Masking Strategy
Armed with the knowledge of these techniques, the next logical step is to implement a data masking strategy tailored to your organization’s specific needs.
An effective strategy begins by auditing your systems, classifying sensitive data, and profiling usage patterns. This shows where and how data masking should be applied. The optimal technologies can then be selected, balancing security, functionality, and cost. Let’s discuss best practices for implementing an effective data masking strategy tailored to your organization’s needs.
The key steps are:
- Data discovery and classification: The first critical step is auditing your systems to identify and classify sensitive data. This allows you to understand what needs to be protected and where.
- Data flow analysis: Next, analyze how sensitive data flows between systems and databases as well as what applications, reports, and masking blindspots currently exist.
- Risk assessment: Conduct a risk analysis by correlating the identified sensitive data with relevant threats. Consider risks like data theft, unauthorized access, and non-compliance.
- Define data masking requirements: Based on the data classifications, flows, and risk analysis, define specific requirements around data masking objectives, acceptable visibility, and usability for different data types.
- Evaluate and select masking techniques: With the requirements established, the most suitable data masking types and techniques can be selected. Consider aspects like existing infrastructure, cost, performance impact, and regulatory needs.
- Implementation: The chosen masking solution is configured, integrated, and rolled out across prioritized systems and databases, based on sensitivity. Proper testing is critical before going live.
- Compliance monitoring: Continuously monitor masked data across environments for compliance with regulatory policies like GDPR. Ensure proper controls for authorized data access.
- Maintenance: Maintain the solution by keeping abreast of new vulnerabilities, updating masking logic for new data types, and optimizing performance.
This structured approach ensures a data masking strategy tailored to your unique environment and needs while delivering maximum security and performance.
The Future of Data Masking
As we venture into an increasingly digital future, understanding and implementing advanced data masking techniques is non-negotiable. Data volumes will continue to explode, especially from emerging technologies like AI and IoT.
Meanwhile, cybercriminals are leveraging increasingly potent attack vectors, from malware to quantum computing. Data masking must constantly evolve to stay relevant.
Exciting innovations on the horizon include intelligent auto-masking algorithms and quantum-resistant encryption. However, these advancements also raise ethical dilemmas regarding privacy versus utility.
Ultimately, a layered “defense-in-depth” approach across people, processes, and technology is key to using data while ensuring its integrity and confidentiality. Data masking forms the core of this robust cybersecurity posture.
Frequently Asked Questions
Does data masking affect performance?
While masking was bandwidth-intensive in the past, modern solutions are highly optimized. Properly implemented masking causes minimal latency.
Is data masking compliant with regulations?
Yes, data masking facilitates compliance with regulations including GDPR, CCPA, and HIPAA by enabling secure data use.
Can masked data be reversed?
Basic encryption is theoretically reversible but more advanced techniques like tokenization effectively prevent de-masking.
Conclusion
Data masking is now an operational necessity rather than a regulatory checkbox. With new data protection regulations on the horizon and threat actors growing sharper, organizations must integrate robust, advanced masking techniques across their data landscape.
A proactive masking strategy coupled with multilayered cybersecurity is key to navigating the turbulent waters ahead.