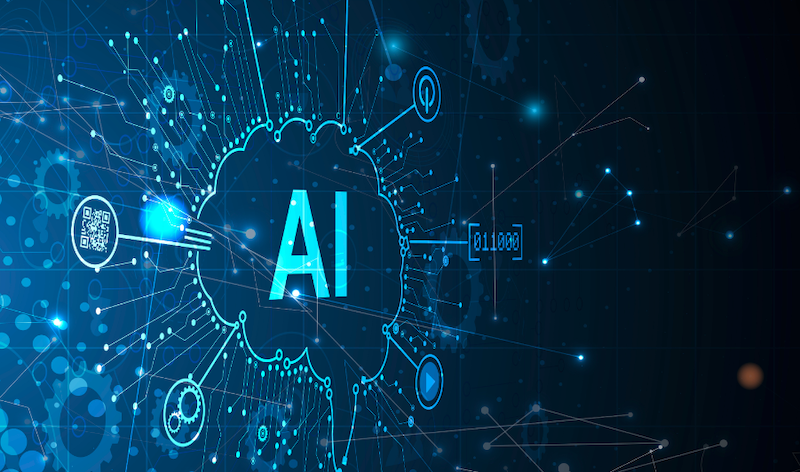
How Machine Learning Helps Improve Fleet Safety
Artificial intelligence is one of those technical buzzwords that has captivated the press and is being discussed in practically every facet of life.
A subset of artificial intelligence known as machine learning, in particular, may assist building and construction fleet operators in optimizing fleet performance while preserving safety as a primary concern.
As roads become increasingly dangerous, safety directors face a challenging task: sifting through terabytes of information to uncover weaknesses in fleet safety.
As data analytics technologies develop, safety managers must learn to use them to surf the data tsunami, stay afloat, and protect the safety of their drivers.
Artificial intelligence (AI) is sometimes mistaken for machine learning (ML). People frequently confuse or use the phrases interchangeably, although they are not the same thing.
AI entails having a machine perform something that a person would ordinarily do. Machine learning, on the other hand, refers to technology that learns or works out how to accomplish something on its own.
Read on to learn more about machine learning and how it improves fleet safety.
What is Machine Learning?
Giving computers data and letting them learn for themselves is exactly what machine learning entails. This system can take a massive quantity of information and develop models based on behavioral patterns. They can learn and improve themselves autonomously by studying known occurrences without being trained. As the system develops, it may be fed fresh data and used to forecast the outcomes of future occurrences.
Machine learning in fleet management enhances how data analytics systems handle large amounts of data. The system begins to learn which data is most frequently reviewed throughout daily use and adapts itself in real time based on the owner’s behavior.
Machine learning algorithms enable the building of dashboards with features that make it simple to examine different data points such as vehicle downtime and specific driver behavior that should be rectified. These advanced neural networks can warn drivers when their vehicles need repair or are going to experience mechanical troubles.
Smart fleet management systems are better capable of diagnosing problems than traditional ones. A company can make better overall judgments when it can view the large picture of its fleet in one location. ML and AI technologies can drastically reduce total expenses.
What Are the Benefits of Machine Learning in Fleet Management?
Machine learning is beneficial in all aspects of fleet vehicle management, including efficiency and safety. Manual methods made fleet management laborious and difficult, but machine learning helps simplify operational operations, making them easy and simple.
Moreover, when integrated with machine vision, ML may improve fleet management even further.
Listed below are a few advantages of ML-driven fleet management:
- Vehicle safety and improved driver
- Fleet operation is visible 24/7
- Dispatching is significantly improved thanks to automation
- Control fuel consumption
- Overall productivity is increased
- Real-time vehicle tracking
- Maintenance cost for vehicles is significantly reduced
- Vehicle routing is improved
- Enhanced the overall productivity
How ML Helps Improve Fleet Safety?
In fleet management, machine learning employs predictive analysis to avoid probable accidents and notify at-risk drivers. A large and full set of historical data may be used to develop a prediction model. It entails examining the actions that led to the accidents.
Using the correct machine learning technology enables risk minimization, accident avoidance, and insurance claim reduction. Understanding, selecting, and then executing the best solution is important to make an accurate and effective forecast.
Integrating centralized data management software with specialized tools allows for rapid information collecting, prediction, and exception handling.
Listed below are a few ways how ML improves fleet safety.
Risk managed with the help of technologies
Machine learning has enabled fleet managers to become more proactive in risk management. Early safety measures were quite reactive. They may have detected things like harsh braking circumstances, but they lacked information about what caused them.
Machine learning goes beyond merely logging such occurrences. It may, for example, include a video to demonstrate what caused the brakes. This reduces “false alarms” and enables fleets to allocate precious resources where they are most needed.
Drivers being vindicated by technology
From time to time, police reports are not always reliable after an accident. When cameras were first employed, it became clear that most of the information in police reports was incorrect. When cameras were put at various transportation businesses, it wasn’t long before the technology vindicated a driver who had been wrongfully accused of causing a crash by law police.
Fleets that use the most up-to-date safety ML technologies can be more focused in their training initiatives. This has aided driver turnover. Rather than retraining all drivers in the fleet on backing owing to a rise in backing events, the fleet may concentrate on only those drivers whose conduct puts them at a higher risk of backing issues.
Human Elements
Fleet companies that use ML safety solutions must also keep the human factor in mind. This should be considered as another tool in the toolkit for assisting drivers in sharing the road safely.
People do not trust a corporation that goes too far down the ML route and just tells them to absolutely believe this machine and do exactly what it says. It must be included in a program. ML exists to help humans make the best decisions possible.
Data obtained by safety technology also provides a safety manager with confidence when dealing with driving behaviors. They are not making decisions based on assumptions or insufficient information. Driver scorecards gamified performance, creating a competitive environment that drove drivers to perform at their best.
Fine Level of Data
Fleet management is a critical component of running a profitable business. Since crashes are statistically uncommon, and carriers can’t look at that fine level of data, one can’t make inferences based on what happens in an hour, a day, or even a week. The safety of a fleet may be dramatically enhanced with excellent machine learning in place.
FAQs
Can machine learning solve all fleet management problems?
No, machine learning is not the answer to all the problems in fleet management. It can provide various information to the company with which they can solve problems using a variety of methods.
What factors affect machine learning?
Listed below are some of the factors that can affect machine learning;
- More data
- Parameters of the method
- Data quality
- Features in the data
Does machine learning require storage?
Machine learning training necessitates the storage system reading and rereading whole data sets, generally at random. This implies that archive systems that only provide sequential access techniques, such as tape, cannot be used.